A digital transformation for asset finance
Part 2: Using Machine Learning in the Wild is our second paper on artificial intelligence in the asset finance industry. In our recent position paper on AI, Part 1: Balancing Risk and Reward, we outlined the high-risk, high-reward nature of using AI in our industry, and machine learning (ML) in particular. We discussed why ML can be an excellent choice, but also highlighted the risks of misusing data, and spelled out the challenges inherent in developing a high-quality solution.
In this paper, we show how auto and equipment finance providers can leverage ML and AI techniques to enhance efficiency and improve customer service. With worked-through examples, Part 2: Using Machine Learning in the Wild will help finance providers decide on whether an AI-as-a-Service approach, or one designed in-house. would be best for their business. Furthermore, we discuss how others in the industry are currently engaging with this new field of technology.
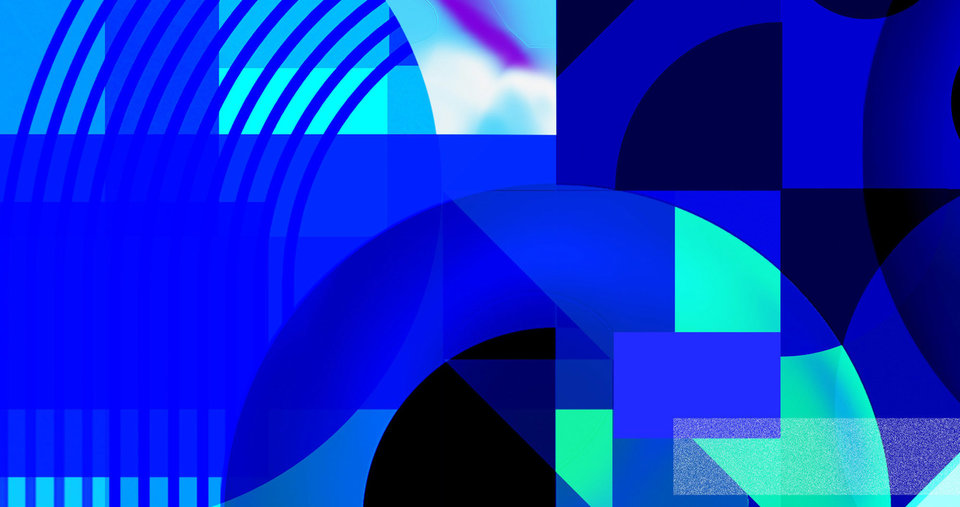
Use Cases
The two use cases outlined in this paper demonstrate two very different approaches to using ML to solve a problem: one that relies on AI-as-a-Service, and one that creates an in-house framework for developing ML models.Automated
Automated object recognition for vehicle licence plates
This use case draws on AIaaS products to integrate licence plate recognition with business logic in Alfa’s own asset finance software platform, Alfa Systems. It demonstrates how these services enable experimentation with ML techniques, at little cost and without requiring great expertise.
End-to-end test analyser
Picking up from our first paper on AI, this use case analyses the results of the automated testing of Alfa's internal source code - this time using our own reusable framework. A neural network trained on historic failures learns patterns between the areas of code that are causing test failures, and the failures themselves.
The experiments detailed in this paper explore different ways to develop ML solutions, and each requires different levels of time, effort and expertise.
However, both solutions rely on domain knowledge of the data used; the first relies heavily on research in object recognition and a resulting dataset that was created by domain experts in that field, while the second is based on data with which most people at Alfa are familiar.
This paper features a foreword from Blaise Thomson, whose speech technology start-up VocalIQ was acquired by Apple and formed an important part of the Siri development team.
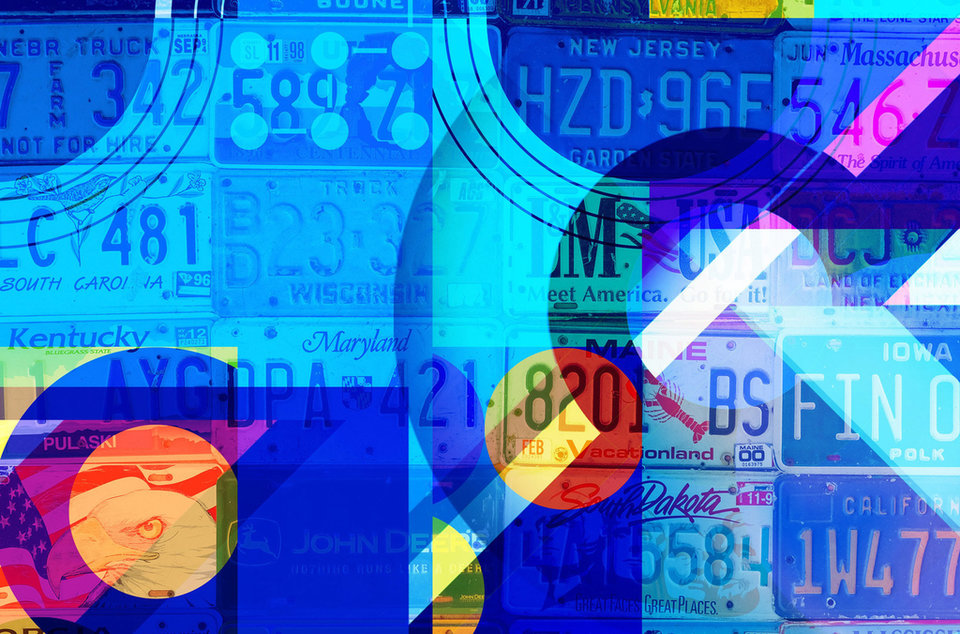
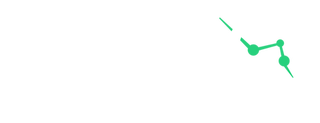
An Alfa and Bitfount company.
The intelligence platform for asset finance.
Bringing intelligence to asset finance. We make access to assets efficient and successful by delivering intelligence to the world’s auto and equipment finance providers. Our models improve credit decisions, delinquency support, fraud detection and more.
Learn more and stay updated at alfaiq.com.