Thought Leadership
Sponsored by Alfa
AI in Equipment and Auto Finance part 3: Moving Forward with Machine Learning
A white paper from Alfa
Alfa has published the third and final paper in its thought leadership series AI in Equipment and Auto Finance.
About the Paper
AI and ML represent an exciting shift for finance providers and, while the benefits are better understood now than they were a couple of years ago, the practical side to acquiring those benefits is still unclear for many. Our aim for this series has been to expose that practical side, and demonstrate where ML can help solve problems and make lenders more competitive.
Part 3: Moving Forward with Machine Learning, produced in association with Alfa iQ, focuses on the trajectory of ML, its uses in auto and equipment finance, and how it will continue to advance in the near future. There follows an in-depth exploration of federated learning, and how organisations can use private data to train ML models without ever compromising the privacy of that data.
Previously in this series
The future of aviation is strictly tied to several factors
Alfa IQ
A partnership between Alfa and Bitfount, Alfa iQ was established to deliver intelligence to the world’s auto and equipment finance providers, with a mission to make access to assets efficient, intelligent and fair.
Alfa iQ will provide industry-leading direction in the use of ML. The partnership brings together Alfa’s industry experience and asset finance data models, with Bitfount’s expert AI data scientists and federated learning platform. Its ambition is to provide the best machine learning models and advanced decisioning scorecards for the asset finance industry.
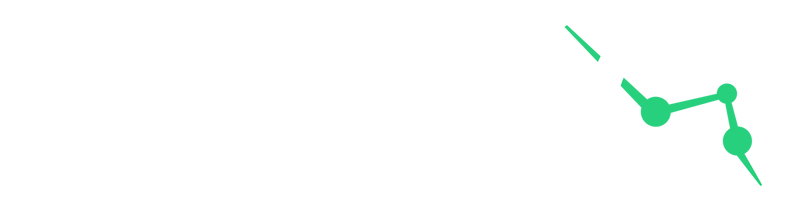
Alfa iQ: The intelligence platform for asset finance. Learn more and stay updated at alfaiq.com.
According to the Author...
Martyn Tamerlane, author of Moving Forward and a Solution Architect at Alfa, said: “Alfa’s aim for this series has been to expose that practical side; to demonstrate where ML can help solve problems and make lenders more competitive, through its ability to detect patterns in vast amounts of data and feed that into higher-quality, sometimes fully automated, decision making. Then, to show ML taking different forms; first as an in-house framework, and secondly relying on AI-as-a-Service.
“Now we consider ML's continued success, particularly in the context of the ever-increasing volume and variety of data that is being collected; but with complex challenges posed by data privacy, fairness and the high level of expertise required to analyse the data effectively. By illuminating the key characteristics of this technology, we’re providing a platform from which people can effect major change.”
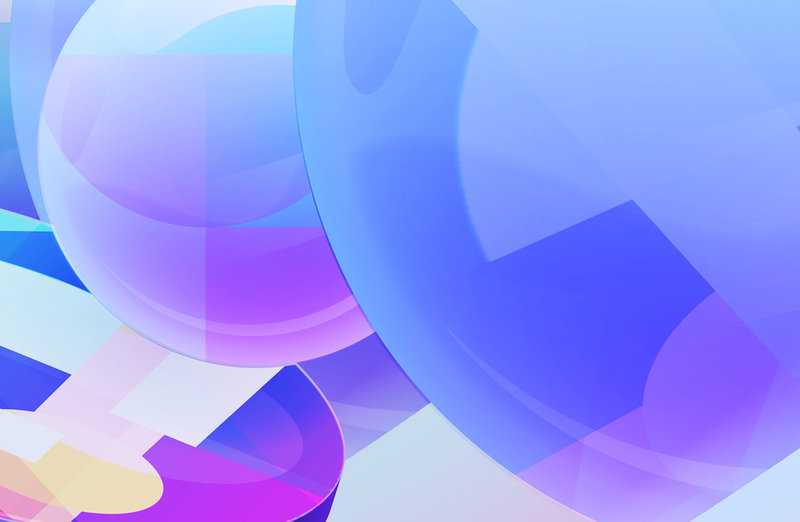
Charting the Meteoric Rise of Machine Learning
In Balancing Risk and Reward, we explored what ML is and why it should be used to solve certain problem types.
But why has ML become so popular in the last decade? Why have we seen so many breakthroughs that make ML relevant for application, such as the production of autonomous vehicles, and ML models that can play games like Go and chess better than humans can?
Better Technology
The computational costs incurred by running ML algorithms are notoriously high. Today, however, we can train an ML model on an average laptop which, ten years ago, would have required a supercomputer.
This is due largely to the rise in Graphics Processing Unit (GPU) capabilities, which happen to excel at the type of operations required by ML data training. As the name suggests, GPUs are designed to render graphics, which require many objects to be drawn on screen in parallel. It wasn't long before this parallel design of GPUs was noticed and repurposed for ML, and in particular the training of deep neural networks, which can be broken down into many independent calculations as part of the training process.
Contact details
Alfa
Moor Place
1 Fore Street
Avenue London EC2Y 9DT
+44 20 7588 1800
Alfa iQ